In today's rapidly evolving world of artificial intelligence (AI), recognizing the concepts of AI bias and hallucinations is essential. These technologies are reshaping many aspects of our daily lives, but they also raise significant ethical concerns that need to be addressed. This article will break down AI bias and hallucination, explain their implications, and highlight their importance in our digital landscape.
What is AI Bias?
AI bias refers to the consistent and unfair treatment that algorithms can show against certain groups or individuals. This bias can surface in different ways, such as higher error rates for specific demographic groups or skewed decision-making processes. AI systems learn from the data provided to them; therefore, if the data contains biases, the AI is likely to assimilate and reproduce those biases in its results.
A critical source of AI bias is historical data. For instance, in a hiring algorithm trained on company data reflecting a history of gender imbalance, the AI may favor male candidates over equally qualified female candidates. A study from MIT Media Lab found that facial recognition systems had error rates as high as 34% for darker-skinned women compared to just 1% for lighter-skinned men. This discrepancy highlights the real-world consequences of biased data on fairness and equality.
AI bias can lead to severe implications in fields like law enforcement, healthcare, and hiring practices. For instance, biased algorithms in criminal justice can cause wrongful arrests, while biased health data can lead to misdiagnoses in underrepresented groups.

Understanding AI Hallucinations
AI hallucinations happen when an artificial intelligence system generates outputs that aren't based on real information or facts. This can lead to bizarre narratives, misleading results, or incorrect predictions. Unlike bias, which comes from learned behaviors and flawed data, hallucinations occur when the AI's reasoning fails, producing implausible outputs.
These hallucinations are particularly concerning in applications like AI-generated text or artwork. For example, an AI might create a convincing story complete with fictional references or images that look lifelike but don’t depict reality. In a notable case, an AI model provided a fabricated quote attributed to a real expert, leading to misconceptions among users who assumed the details were accurate.
Understanding the root causes of these hallucinations—often tied to limitations in model design or insufficient training data—is vital for enhancing the reliability of AI systems.
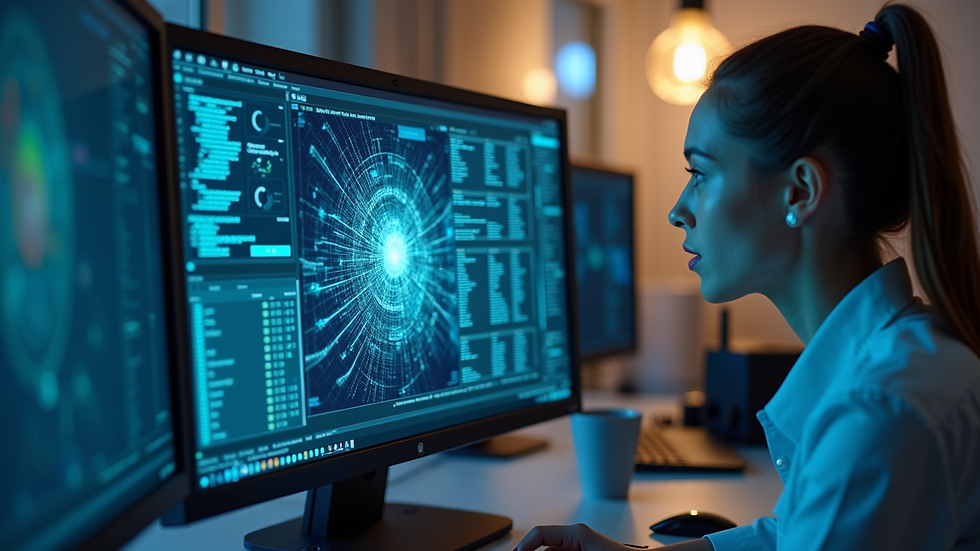
The Implications of AI Bias and Hallucinations
The consequences of AI bias and hallucinations extend well beyond technology. They can influence social justice, economic equity, and even mental well-being. Biased AI can reinforce existing inequalities and foster new ones. In a 2020 survey by Pew Research, 70% of Americans expressed concerns that AI could perpetuate bias in hiring, lending, and policing, highlighting widespread anxiety about the technology's shortcomings.
Furthermore, hallucinations can mislead users, fuelling disinformation and undermining trust in technology. To combat these challenges, developers, regulators, and users must work together. Strategies may include:
Refining Data Collection: Ensuring diverse and representative datasets to reduce bias in AI systems. For example, including varied demographic representations in training datasets can lead to fairer outcomes.
Implementing Evaluation Measures: Regular assessments of AI outputs to identify and rectify hallucinations. Developing AI systems with built-in checkpoints can help catch errors before they reach end-users.
Awareness is the first step toward addressing these critical issues, followed by transparency in the decision-making process of AI algorithms.
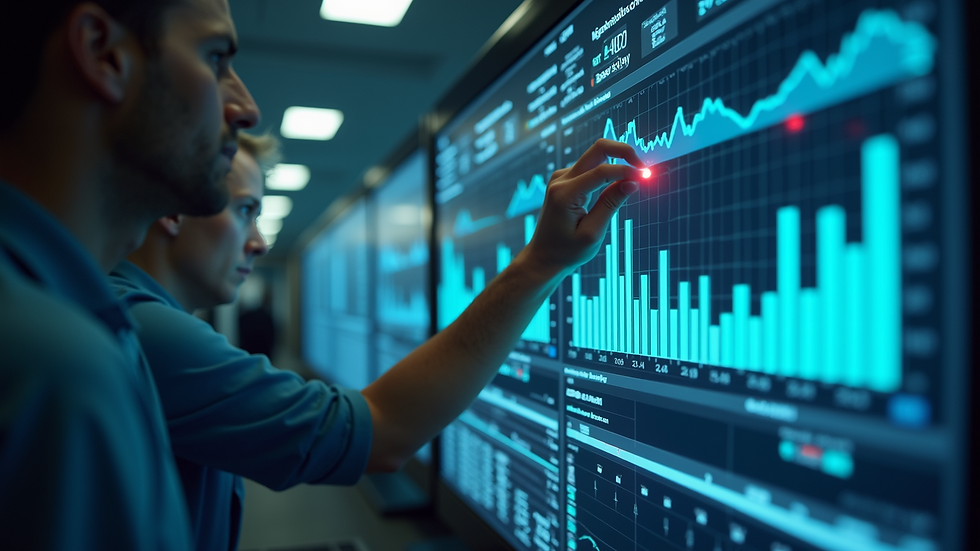
A Vision for the Future
AI bias and hallucinations present significant challenges as we integrate AI more deeply into our lives. Understanding these concepts is crucial for navigating the ethical landscape surrounding technology. Commitment to awareness, transparency, and proactive measures can help mitigate these issues, paving the way for a more equal and reliable digital future.
As AI advancements continue, we must create a space that values ethical considerations and ensures that technological progress serves the greater good. The path toward improved AI is ongoing, beginning with informed conversations and a shared commitment to responsibility.
Comments